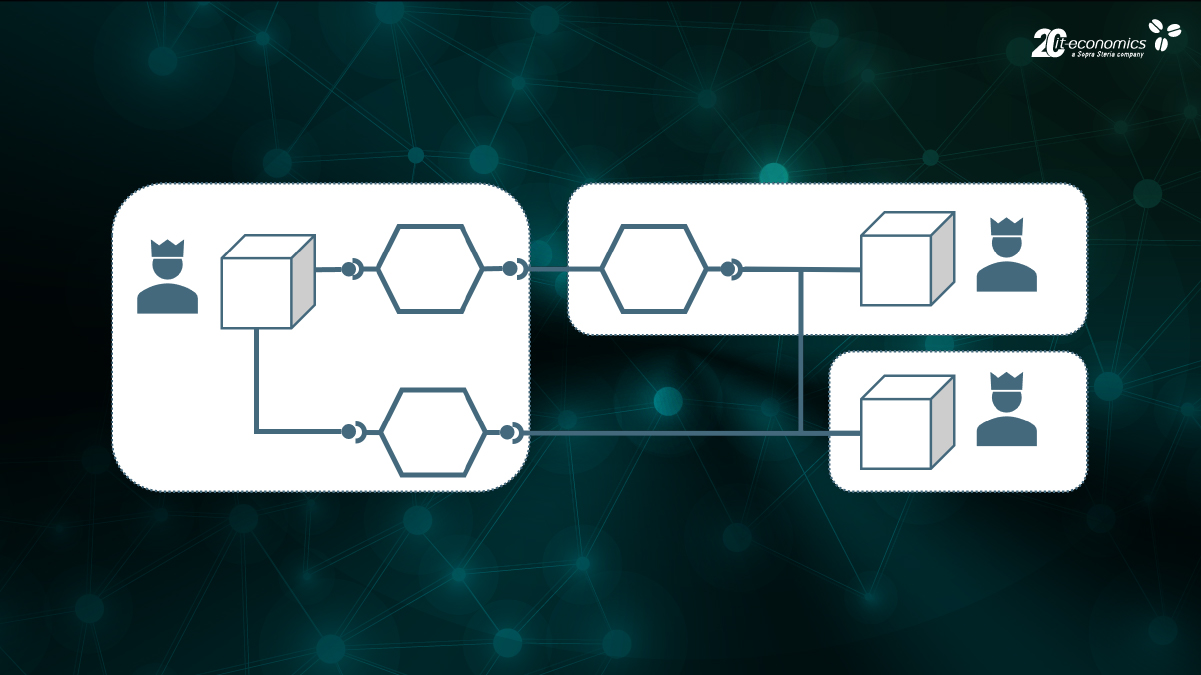
September 2023
data mesh
Data Mesh is currently a much-discussed approach when current architectures and organizational forms for data management in companies no longer work. Behind this lies a socio-technical concept for a new way of dealing with data.
With this blog post I will show you what the data mesh approach is all about and how it meets the challenges of current data warehouse or data lake architectures.
In further blog posts you will learn the first steps, how companies can implement this approach for themselves and also what the start towards data mesh with existing data warehouse or other data management tools can look like.
Challenges of current data warehouse and data lake architectures
Data Warehouse
Data warehouse architectures are characterized by the fact that consistent, high-quality and integrated data are brought together in a central architecture, which can then be used for measurements and analysis. Strategic decisions are then made on this basis.
To create the database, there is a central team consisting of experts in dealing with analytical data. As long as the domain knowledge required to produce this database is limited, this approach works very well and ensures that the data quality is high.
However, we have been observing for several years that the amount, variety and speed of data continues to increase (keyword: big data). This also makes the domain knowledge needed to understand the data increasingly complex. And at the same time, the requirements for the provision of data and data analyzes are increasing, as these no longer only come from management - as in the early days of data warehouses - but data-based decisions are already commonplace in many everyday applications. This is where a central team reaches its limits, as, on the one hand, there has to be increased communication with the experts in the domain in order to use their knowledge. On the other hand, the central team receives many more inquiries and orders to integrate additional data. Both the increased communication and the implementation of the many orders require capacity that only exists to a certain extent in a central team. If further scaling of the central team is no longer possible or sensible, alternative approaches must be considered.
data lake
At the time the term big data was coined, the data lake approach was developed in response. A data lake is a large database in which the available data was initially collected without any demands on consistency, integrity and quality. In this way, it is possible to make data available in large quantities, with a wide variety and quickly. Topics such as data quality, consistency and integration were only focused on later, depending on the need for a specific use case. This approach has advantages if, for example, fast pattern recognition is the goal. With increasing requirements for the traceability of data flows and clear responsibilities for data, for example in highly regulated industries or when it comes to personal or other sensitive data, data lake approaches are also reaching their limits.
The data mesh approach
The data mesh approach now attempts to combine the advantages of well-known architectural approaches and at the same time resolve the problems and limitations described above. The data mesh approach is therefore not a pure architectural concept, but rather combines architectural, organizational and cultural elements.
Below I will discuss these elements:
Redistribution of responsibility
Instead of a central team, responsibility is decentralized and shifts left into the domains. The responsibility for providing data lies in the data mesh, i.e. where the data is created or generated and where the domain knowledge is. At this point, the know-how is also available as to what requirements must be placed on data quality and data security and what constitutes sufficient data quality for this data or what pitfalls arise from inconsistent, incorrect or missing data.
However, to ensure that decentralization does not create strictly separated silos, the redistribution of responsibility with federal governance includes another important aspect. Instead of making specifications from above, it is necessary that the decentralized responsible parties come together and jointly define and commit to specifications.
product thinking
Data is no longer provided “as is” but in the form of products. Thinking of data as a product means, on the one hand, that those who are responsible for providing it keep the consumer in mind and only offer products that they know or assume will meet the needs of consumers and that they will benefit from them can be used by as many consumers as possible. On the other hand, data product also means that what is provided changes. Not only is data provided, but data is provided along with it
- Transformation code that creates the appropriate preparation, aggregation or transformation from incoming data.
- Defined interfaces so that consumers can access the data prepared in this way.
- Service level objectives that determine who can access the data, what level of data quality is guaranteed, etc. These are also created in machine-readable form so that it can be read by the data platform.
platform architecture
The above elements only work well if there is a central data platform through which the domains can develop and deploy their data products. The central platform checks whether the data products comply with generally applicable provisions for provision, as this is the only way to ensure that the data products function as a self-contained component, are interoperable with the other data products and can all be searched for and found in a central location.
In addition, the platform is responsible for ensuring that the service level agreements of a data product specified by the domains are executed automatically. For example, the service level agreement specifies who can have access to the data product. The platform evaluates this information and ensures that retrieval of the data from the data product via the output ports of the data product is only possible from other domains if they belong to the corresponding rights group. In addition, the platform can also note on the data product if it does not correspond to the guaranteed data quality, for example with regard to the timeliness of the data.
Zhamak Dehghani has in yours Book Four principles are defined from these elements that strongly interact with each other. For further basics of the approach, I would therefore refer you to this book.
Starting next week you will find a new blog post in which I will outline the first steps that companies should take to build a data mesh in their organization, including tips on how to build on existing data warehouse or other data management tools. to start with a data mesh.

author
Dr. Saskia-Janina Untiet-Kepp
Senior Manager
You must view the contents of reCAPTCHA load to submit the form. Please note that data is exchanged with third-party providers.
More information